The destiny and manifestation of Heidegger's "technological thinking" through AI
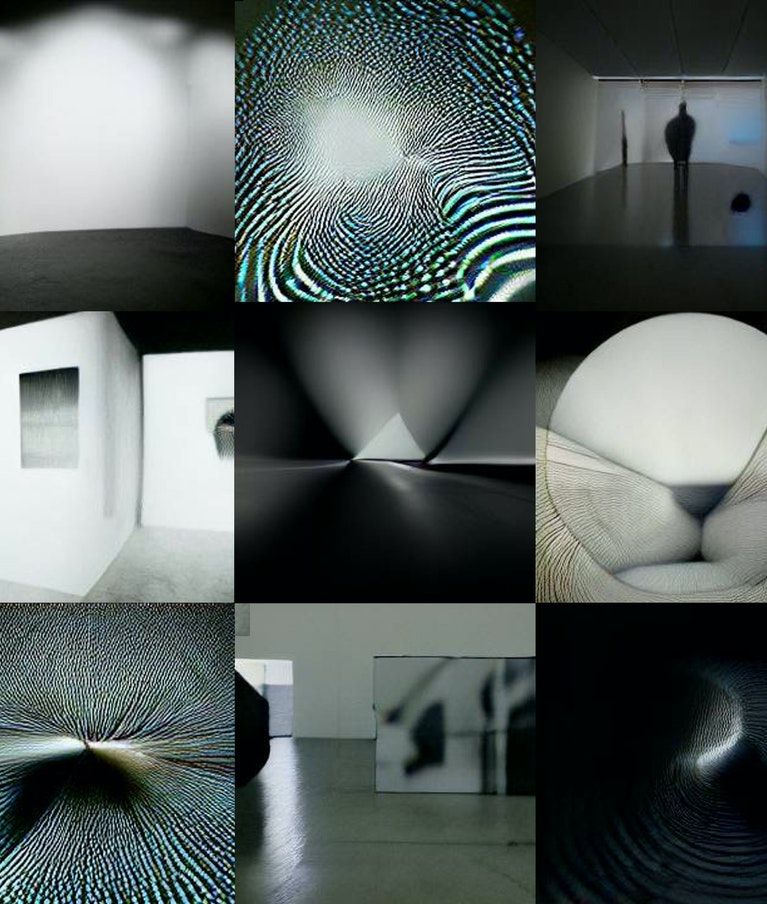
It all started one fine morning when I was discussing the work of Martin Heidegger with a friend regarding his "question concerning technology". Now Heidegger is unique as a philosopher as rarely there is a convergence of Computers and Philosophy. He was resurrected and put back into prominence in the debates of Artificial Intelligence by Hubert Dreyfus through his paper titled "Why Heideggerian AI Failed and how Fixing it would Require making it more Heideggerian"
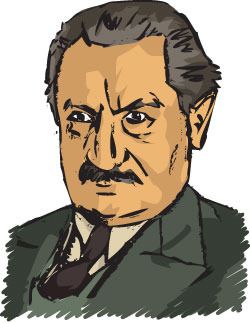
His uniqueness stems from his positioning in history I reckon. While Kierkegaard, Kent, Nietzsche and all the other Eastern philosophers or the ancient Greek philosophers dealt with a myriad aspects of life and metaphysics, they all lived in a timeline where technology played little role compared to what had to come. Heidegger however, saw the World War, which pushed forth the rate of technological advancement many fold. The Radar, Atom Bomb and the entire modern computation technology as pioneered by Alan Turing came forth during the war. He witnessed it first-hand. Hence the question regarding technology became so prominent in his works for he must have witnessed how the history and future of humankind shall be decided based on the technological prowess of nations. We thought he dwelled on this aspect deeply. And he really did.
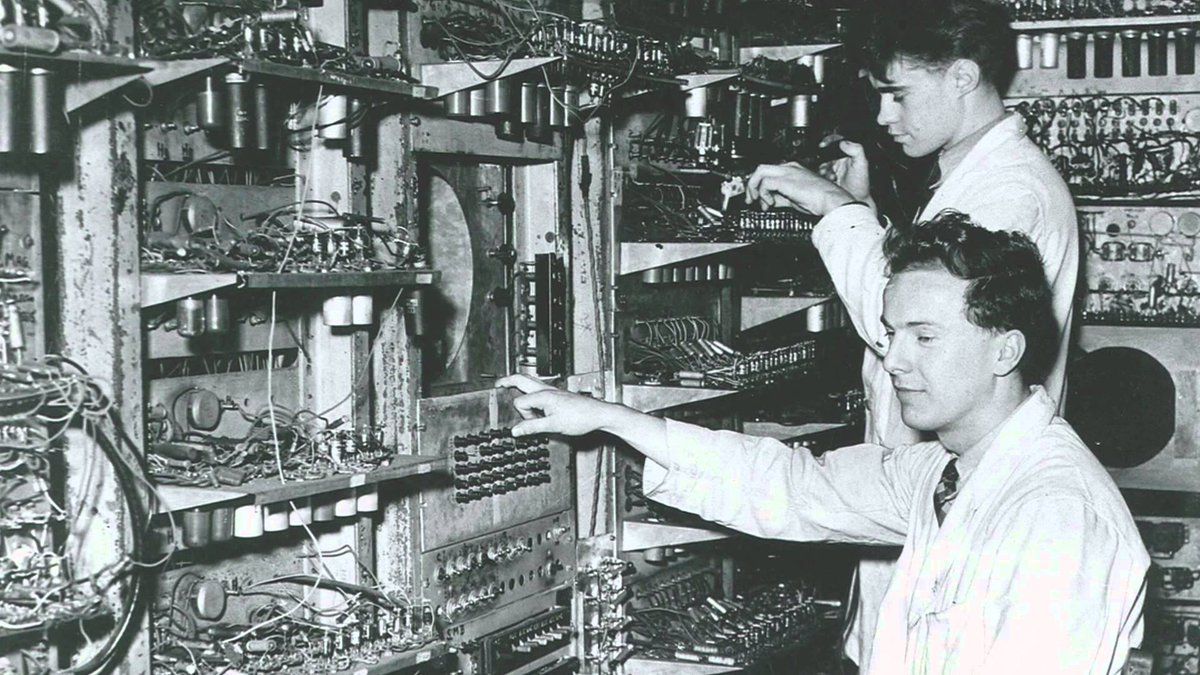
He seemed to have grasped the duality a technological advancement posed to humanity. The questions dwelled upon by him touch very significantly, among other practical aspects of modern day living, topics such as global warming and ecological conservation as evidenced by his notion of "receiving sky as sky". You can research about that topic which is fairly involved and beyond the scope of this writing.
Heidegger witnessed how the history and future of humankind shall be decided based on the technological prowess of nations
One other aspect which naturally conjoins itself to his "question regarding technology" and a certain kind of "technological thinking" is the advancement of the modern day AI. It is a perfect example of how he claimed the "poetic" would be deserted from human life as would be the "mysterious".
He was a kind of a moderatist it appears. He did not advocate stopping the advancement of technology. Infact, he did believe it was the ultimate manifestation of what he called "interpretation of the metaphysics". A kind of clearing which he called as destining for the humankind. So he embraced technology. But at the same time he was worried that it would also become the predominant way of "being". And it would bring in some detachment from the synthesis of essence of "being". Hence he propagated the ideas of receiving "divinities as divinities".
He wanted to preserve the "poetic" and the "mystical" in life — which he believed — is what the modern day technological thinking would impede. And that is a real question posed by the excesses of AI in future which humanity should be wary of. As opposed to what a lot of people claim a sentient AI would pose. Because a sentient AI is not a construct staring at humanity today by a very long margin. But an alienation of "being" from "natural living" as discussed by Heidegger in his "question concerning technology" and the loss of the poetic in that context as surmised by him seem to be elements an excess of AI may eventually bring about in our lifetime.
An alienation of the being from natural living as discussed by Heidegger in his "question concerning technology" and the loss of the poetic in that context as surmised by him seem to be elements an excess of AI may eventually bring about in our lifetime.
What took the AI revolution so long?
At the dawn of the field of artificial intelligence, few of its pioneers famously predicted that solving the problem of machine vision would only take a summer. We now know that they were off by half a century. More recently, Elon Musk in a 2015 interview with Fortune magazine said Tesla vehicles will drive themselves in two years. We hope he does better.
This then led us to ponder: the promise of AI is immense as we understand. We have first hand witnessed the power of ML and AI manifest beautifully in our daily lives and at work. Google search and Netflix being just a couple of example of AI touching our lives everyday. If we take a cue from Andrew Ng, AI is going to be the next electricity.
Just as electricity transformed almost everything 100 years ago, today I actually have a hard time thinking of an industry that I don’t think AI will transform in the next several years. - Andrew Ng
It is if we take a step back, a big promise and potential to manifest. And Heidegger would just point that the technological thinking grasping the human consciousness would definitely entail such a play in its eventuality. But this brought forth another question.
The pace at which AI has yet been applied is dismal for such a predestination. There has been a lot of work we realized which has been done in the theoretical constructs, but the real life impact has been slow to manifest itself.
This felt like a very interesting proposition to explore within the constructs of Heidegger's proposition of technological destiny of mode of "being" for humans which seems to aptly come about in AI as a construct. And also the proclamation of it being the equivalent of the force of electricity for the modern world in terms of its impact potential. And that got us to dig deeper at the question as to how the promise can be achieved
The Devops Culture
I remember the time I was leading a critical project at one of the largest retailers in the valley. The engineers were moving quickly to ship new features for the upcoming Holiday season. Technical debt was compounding but ignored. We had some of the best engineers but the team was nervous as the holiday approached. The flash sale went live and within seconds the entire site came crashing down. In those moments it was easy for me to connect the dots backwards and that is when I thought; Software Development and Deployment of code has to be rethought if we have to deliver quality at scale and speed.
Life can only be understood backwards; but it must be lived forwards. - Soren Kierkegaard
The single biggest impediment in the delivery of quality software is the siloed divides that exist between the different teams. A key first step involves a closer collaboration and a shared responsibility between development and operations teams for the products they create and maintain. This helps companies align their people, processes, and tools toward a more unified customer focus. Once the cultural shift is accomplished, the focus should be on automating every piece of the software delivery lifecycle.
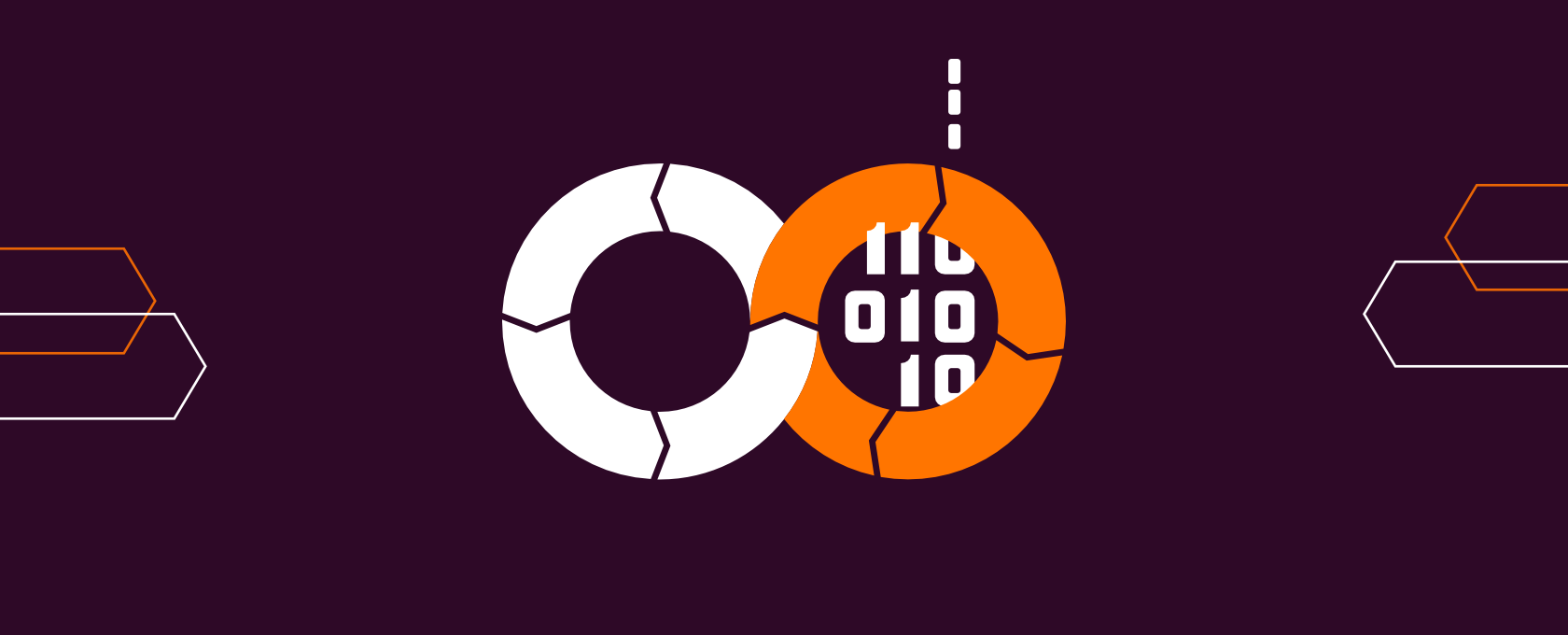
A big mistake teams make is to think of DevOps and CI/CD as just automation. Without the right mindset, rituals, and culture, it’s hard to realize the full promise of DevOps. At the heart of the DevOps culture is increased transparency, communication, and collaboration between teams that traditionally worked in siloes. A mature DevOps team embraces a customer first mindset and works backwards to ensure they have a pipeline streamlined to deliver value to them at speed and scale. Today efficient teams the world over, use those basic principles and the tools and infrastructure associated with it to transform the world around us using technology at a breathtaking pace.
A big mistake teams make is to think of Devops and CI/CD as just automation.
In my view the absence of DevOps culture is precisely the reason why we have not been able to bring about an AI based transformation in our lives which has been promised for so long we argued. The theory and algorithms have been built, but the engine for enhancing the pace and deployment is not good enough. To give a vague corollary which connects to the metaphor of electricity as professed by the esteemed Andrew Ng, the electricity production has been done-with, be in a hydroelectric plant or a thermal one, but the grid needs to be established. And if we really needed to romanticize the construct, a Nikola Tesla needs to find with his team a way to distribute the electricity to the masses in the form of AC which is cheaply done-so enough to make it a technological force Heidegger would be proud of.
Now we may never have the prowess of a Tesla individually, but we can begin to, as a collective, build the general infrastructure that disseminates the AI as he did for electricity. And hence started our little project to build that neuron-highway or the neuricity grid, with the components needed to realise the cultural transformation and streamlined operation necessary to deliver to the people on the promise of AI. The DevOps for AI and ML piece, or MLOPs. And more.
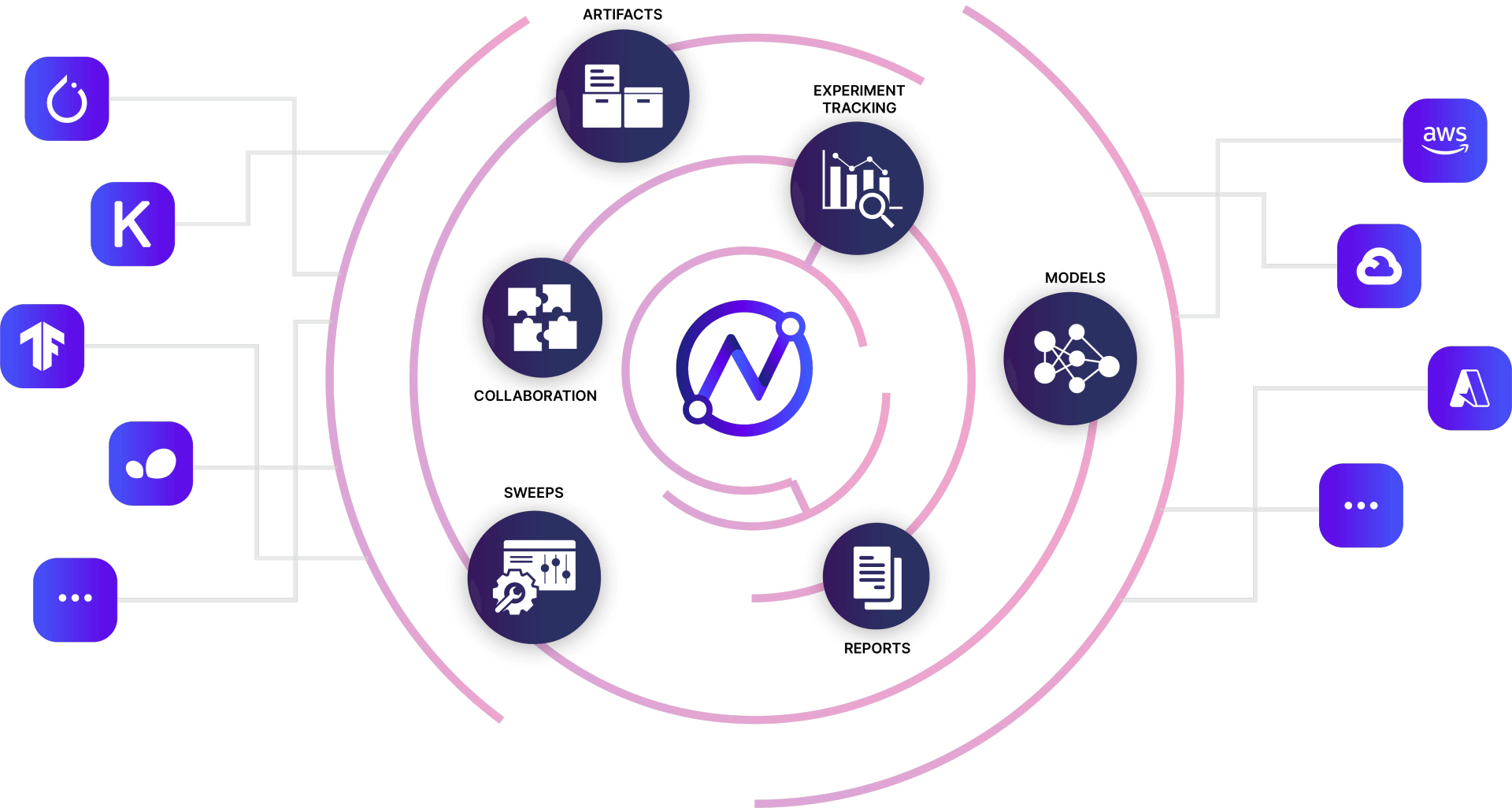
At its core, the Newron ML platform aims to break the siloes that exist in the lifecycle of ML model development and delivery. In our first version, we are offering following capabilities and will continue to build from there.
Experiment Tracking - Automatic experiment tracking for machine learning with tools to version datasets, debug and reproduce models, visualize performance across training runs, and collaborate with teammates.
Hyperparameter Optimization -
Artifact Versioning - Datasets and model versioning that allow for easy reproducability.
Model Registry - This allows to track models ready for deployment.
Reports - Publish and collaborate with team or the wider community.
The Production and Distribution Grid of AI
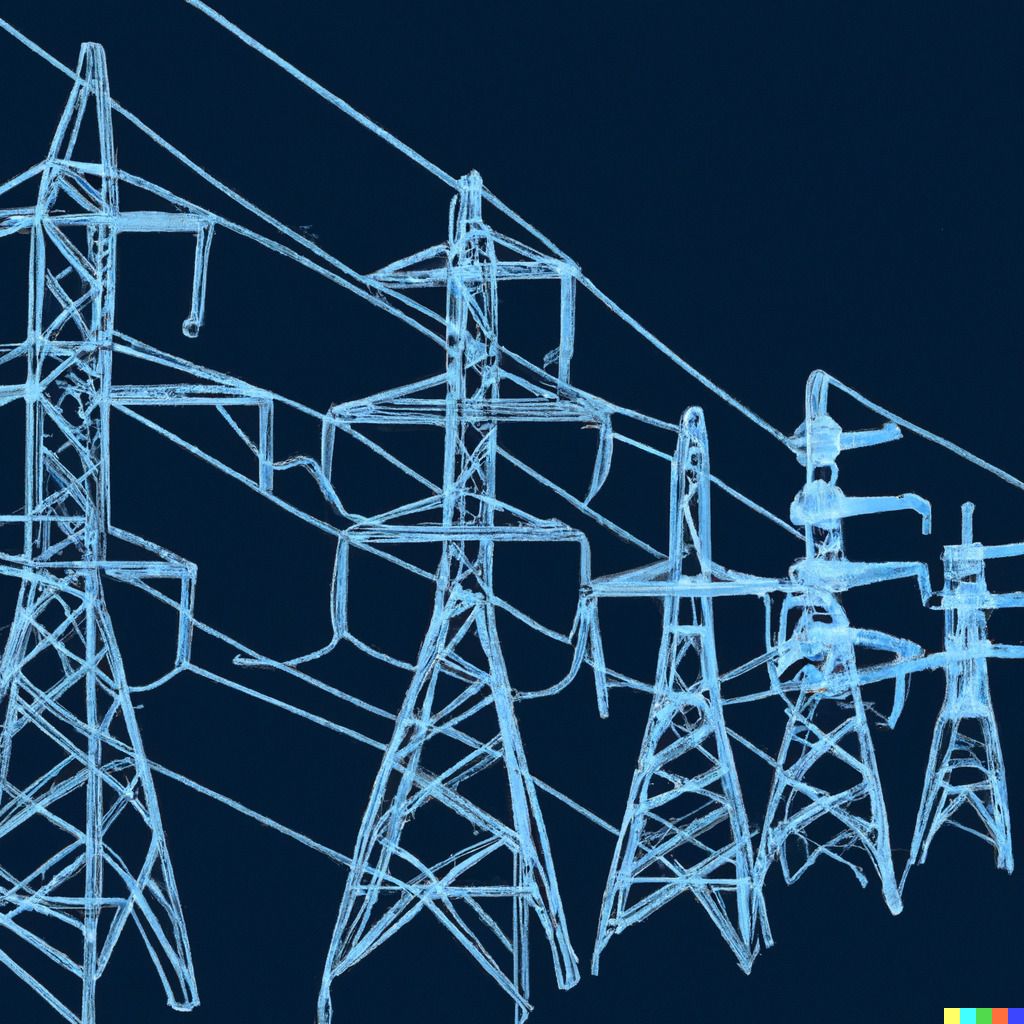
Once we have defined the mission statement of "re-electrification" of the modern world through AI, we ought to build the infrastructure for its deployment as we discussed. That is to say, the equivalents of the power plants and the distribution grids. The last decade in my view has been about building the power generation systems. The Machine Learning technology with the models, algorithms and the curating of the associated data and systems around it. But all of this is done now in siloes. People have tuned their models perfectly in their algorithmic nuances and put them forth on platforms like the kaggles of the world. That is a great achievement. But as pointed out by many practitioners, now is the time to make those models work with the data-set. And beyond. This is all the discussion around the data centric AI and the road ahead.
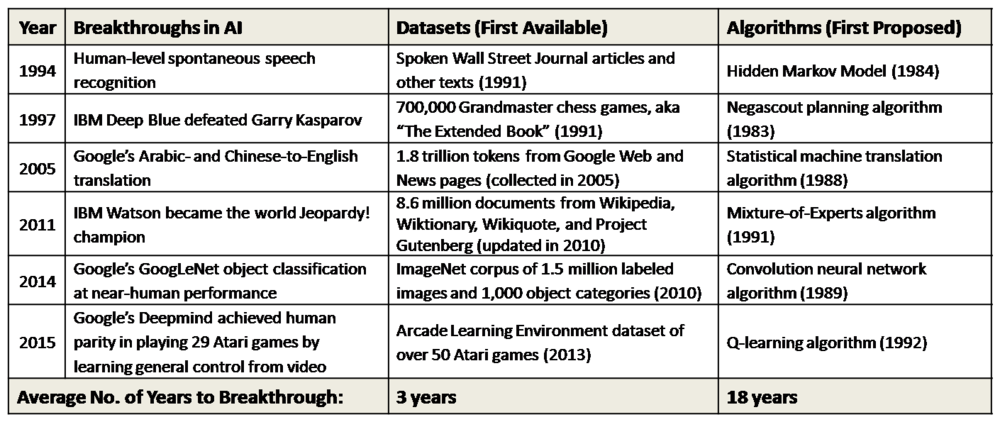
And there has been good progress in that area with the proliferation of tools like various data labeling and data augmentation platforms. These ML labeling and annotation systems have already revolutionized certain aspects of the proverbial grid and made a difference to the business end of things. Take for instance the impact of the annotation and labeling piece on e-commerce. All the major players in the space globally have dedicated processes and systems in place for these to enhance efficiency and productivity. And that has shown an impact of ML on the bottom-line.
It is high time we enhance the infrastructure to make similar impact on the other aspects too. Take for instance the model accuracy and deployment. Today, using a tool like newron, an ML engineer can improve her model much quickly by using experiment tracking and seamlessly visualizing each of the runs. Newron helps not just in experiment tracking with different runs but also helps deploy those ML models easily in the production environment. In production, Newron monitors the models to track the key indicators to provide feedback loops and alerts. And the scope doesn't end here.
Infact, this is just the start of the development of the distribution grid to fast deliver the AI to impact business in tangible ways. There are tenets of CI/CD and agile code development which shall be applied to the whole Machine Learning flow. For instance, we can better integrate the raw data with the grid and connect the labeling tools with the ML deployment engine. There are so many subsystems which make the process very slow today which shall be integrated in a click of a button way to make the process faster. This and many more subsystems form the pipelines of the AI flow plumbing system we aim to deliver at newron.
The Asphalt that lays ahead
It is easy to lose sight of the bigger picture in the intricacies of the work today in a field like ML and AI. The rigour of the models and the Neural Network layers is involved enough to engulf the energy & time of the practitioners. But, if we were to believe some of the bodies involved in the governance side of things working for a while now from the SF bay area, a new picture emerges for the future. One which we concur with in a vast degree.
One such area of interest is governance and responsible AI, or the DaSein AI in a Heideggerial context if we may. We have to understand that ML and AI are decision making systems as opposed to traditional software. And there are a lot of datasets involved. As the applications would become more and more widespread, companies and governments at both state and local levels would start to get impacted. And the argument goes right from payment certifications to National Security issues then. And suddenly the macro would start to dictate the the narrative much more than the micro. And that motion is already set we believe. The majority of the asphalt is yet to be laid for future. The more agencies and organizations that start to get involved in this for future, the bigger the ML Ops and maybe the AIOPs systems would become. It shall encompass all category of software, right from dala piloting to cloud infrastructure and also hardware. The model training in local or customized GPU, CPU or TPU would not always work in the deployment systems which can be on premises and even hybrid systems depending on security and governance creation. The folks at some of the think tank bodies in this area forsee a lot of disruption even in the hardware systems to enable such a widespread adoption on a customized basis. Actually they cite that Apple has already started to make investments in that area. There shall be as many applications of the customized dataset-model combinations as shall be the variety of data with the bias. And since we are talking about decision-making and automated workflows across the board, the governance or ethics piece, or the DaSein AI aspect shall keep enhancing. And this makes this a massive opportunity of investment for the Venture community as the market size of such a paradigm would be gargantuan. And the odds are much in favor now.
The Final Frontier
The culmination point of all the above sub-facets is the impact that it shall be able to create on business and government agencies. Going back to the original electricity analogy, it was a revolution of power distribution because the technology was able to be finally mass produced and distributed at a cheap price-point. So while there is one aspect of identifying the businesses and sectors where the first adoption shall occur to make it a feasible outcome, there is another to make it efficient and optimized on the cost front. And sometimes, that would be the difference in the viability and closure of a project.
Even to understand the whole price dynamic, we would need to do some actual dip-tests which shall be costly. And a certain percentage of those would turn viable. Today, very few business propositions are being tested compared to the enhancement done in the model front. And that is the reason, a business leader is always in a state of second guessing the scope and viability of the AI projects they oversee. There is less than ideal level of metrices and reports that they get, to track the progress and the performance of the project systems they have initiated.
All of this is because the teams work in siloes and there is no pattern and reproducible process which makes the process more understandable and predictable for the leaders. We need to have systems which help leaders in tracking, visualising, forecasting and then measure the performance of teams with well defined metrices. In addition to the ML engineers who have started to incorporated the rigours of model experiment tracking and analysis now. That is going to be the difference between an academic exercise and a pointed ROI driven business application. And that is the promise a well-laid grid such as Newron holds for the business leader and the AI practitioner. To bring the technology out from the lab and into the real world. To re-electrify the world through AI by making the system simple and very accessible.
Maybe it takes the will-to-power that Neitzche, or a Knight of faith that Kierkegaard spoke of, a saadhana of a artist as practised in the east, but the world is overdue its Heideggerian AI as promised and we all ought to assert ourselves to that end we believe. Please join us in our adventure to unravel the mystery of AI based re-electrification of the world.